Production rate of 200%! The eight directions of "AI+fermentation" include multimodal fusion, multi temporal prediction, millisecond level control, and digital twins ..
AI will integrate synthetic biology, participate in strain screening and process design, and move towards the era of "intelligent biological factories".
1、 Full parameter sensor network deployment
Deploy high-precision sensors such as temperature, pressure, and dissolved oxygen at key locations in the bioreactor, build a real-time data acquisition network, and generate millisecond level data streams.
2、 Data preprocessing and anomaly detection
Filtering sensor noise through noise reduction algorithms, combined with outlier detection models to identify equipment faults or data anomalies, ensuring the reliability of input data.
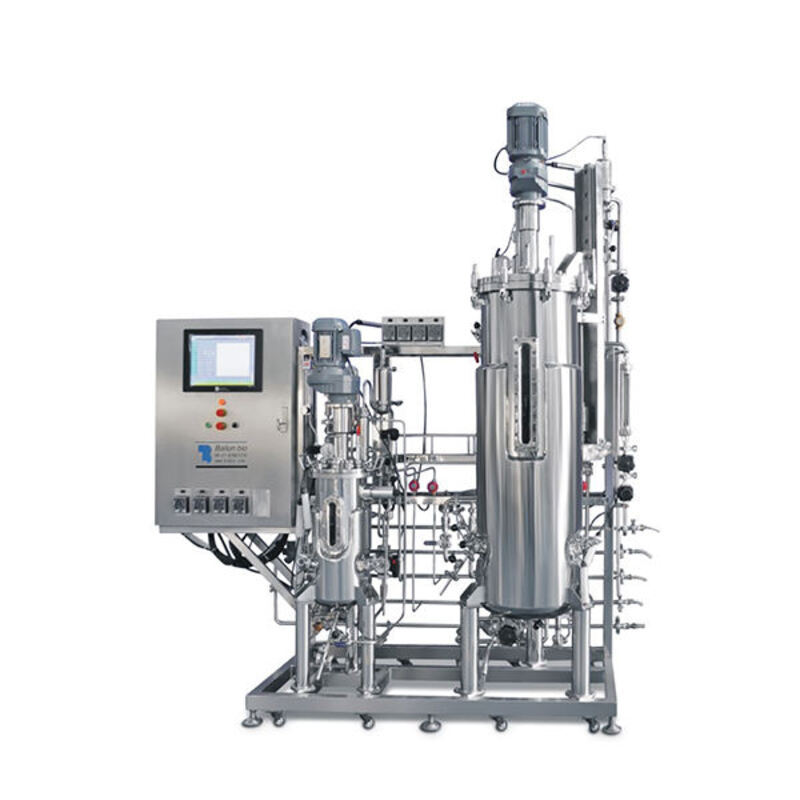
3、 Real time mapping of digital twins
Based on historical data and physical models, a digital twin of a bioreactor is constructed, combined with CFD and deep learning, to map the internal flow field, mass transfer, and metabolic processes of the tank in real time, and predict the impact of stirring rate on mass transfer efficiency.
4、 Multimodal data fusion analysis
Integrating multi-dimensional data such as sensors, images (cell morphology), and spectra (near-infrared spectroscopy) to improve monitoring accuracy.
5、 Multi step time series prediction model
Using models such as LSTM and Transformer, taking the fermentation process as an example, inputting current parameters can predict substrate consumption, product synthesis curve, and feeding timing for the next 48 hours, generating time node operation plans.
6、 Millisecond level closed-loop control technology
Establish a Model Predictive Control (MPC) framework, update control strategies in milliseconds, dynamically adjust parameters such as stirring speed and ventilation rate, and control dissolved oxygen fluctuations within ± 2%, which is superior to traditional PID control.
7、 Intelligent warning for abnormal working conditions
By using Gaussian mixture and other models to learn the normal parameter distribution, a three-level warning is triggered when the dissolved oxygen drops by 15% and the pH drops by 0.5, which shortens the response time and ensures production safety.
8、 Intelligent optimization of process parameters
Using deep learning to optimize replenishment strategies; Quickly adapt to new scenarios through transfer learning, reducing process debugging time.
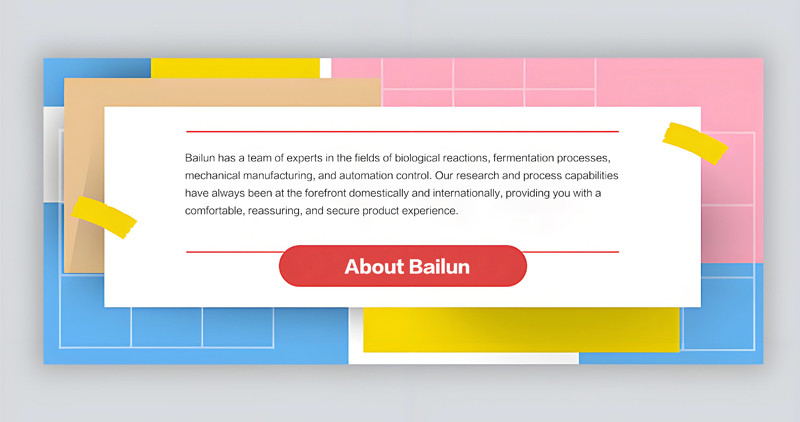